Introduction to Malware Binary Triage (IMBT) Course
Looking to level up your skills? Get 10% off using coupon code: MWNEWS10 for any flavor.
Enroll Now and Save 10%: Coupon Code MWNEWS10
Note: Affiliate link – your enrollment helps support this platform at no extra cost to you.
As criminal tactics become more sophisticated, the challenge for law enforcement isn’t just to solve cases – it’s to predict and prevent them.
AI-powered predictive analytics is key to staying ahead of criminal activity in 2025.
Nearly half of all law enforcement agencies surveyed by Cognyte view predictive analytics as a game-changer for accelerating investigations in 2025. The survey shows predictive analytics are a critical step to overcoming police force challenges posed by a digital transformation in criminal activity.
In this blog, we’ll explore how predictive analytics tools and techniques are shaping policing and why the right solution is essential for turning insights into action.
What is Predictive Analytics?
Predictive analytics converts vast amounts of historical data into actionable insights, using statistical algorithms and machine learning to anticipate what’s likely to happen next.
Imagine a city facing a surge in human trafficking cases. A law enforcement analyst could use predictive analytics to uncover patterns in victim reports, transportation routes and online recruitment tactics. By analyzing data points such as incident locations, victim demographics, social media activity and financial transactions, they could identify hotspots and predict trafficking operations.
For example, by correlating hotel booking surges, spikes in ride-hailing activity near event venues, and illicit online ads, the analyst could detect organized networks exploiting large gatherings. These insights would help law enforcement allocate resources strategically, disrupt trafficking operations, and collaborate with NGOs to support survivors
There are four core elements of predictive analytics tools in law enforcement. They are:
1. Data Collection & Preparation
- Collecting, cleaning and structuring data from crime reports, digital evidence, surveillance feeds, social media, and public records.
- Ensuring data quality and accuracy to generate reliable investigative insights.
2. Modeling (Algorithm Selection & Training)
- Applying machine learning and statistical models to identify crime patterns, suspect behaviors, and high-risk locations.
- Training models on historical case data, financial transactions and other investigative sources to predict future incidents.
3. Evaluation & Validation
- Testing model performance using crime detection accuracy, anomaly detection rates and investigative relevance metrics.
- Ensuring that predictions are actionable and reduce false positives before being used in investigations.
4. Deployment & Monitoring
- Integrating predictive models into law enforcement platforms to support real-time decision-making.
- Monitoring and refining models based on new crime trends, emerging threats and officer feedback to maintain accuracy.
Applications of Predictive Analytics in Law Enforcement
Here are some real-life examples showing how law enforcement agencies leverage predictive analytics to fight crime, disrupt threats and optimize resources.
1. Forecasting crime hotspots with predictive analytics
By analyzing crime reports, geo-spatial data , and online discourse, predictive analytics tools can forecast high-risk areas and identify patterns linked to increasing violence or property crimes.
Example: Law enforcement agencies are increasingly adopting predictive analytics to combat Grand Theft Auto (GTA) crimes. By analyzing crime data, vehicle theft reports and geospatial information, these tools help identify patterns and hotspots for vehicle theft incidents. Analysts can examine variables such as the types of vehicles most frequently stolen, the time of day thefts occur, and the methods used to bypass security systems.
For example, a sudden surge in luxury vehicle thefts within a specific neighborhood might indicate a well-coordinated theft ring. By correlating this data with surveillance footage, license plate recognition system hits, and even social media chatter about stolen cars, analysts can pinpoint areas where thieves are most likely operating. These insights enable law enforcement to deploy proactive patrols, coordinate with car manufacturers for advanced anti-theft measures, and warn residents about emerging trends in auto theft.
2. Detecting fraud and financial crime with predictive analytics
Fraud detection has advanced with predictive data analytics, allowing agencies to:
- Monitor financial transactions for anomalies linked to organized crime and money laundering
- Detect fraudulent patterns in real time, helping financial crime units respond before massive losses occur
- Uncover links between criminal networks, identifying suspicious entities across multiple financial institutions
Example: Predictive analytics have been used to identify and dismantle fraud networks by uncovering hidden connections between suspicious transactions, shell companies and high-risk entities across multiple financial institutions. By analyzing patterns and anomalies in transaction data, law enforcement can prevent fraudulent activities. Additionally, these analytics enable investigators to prioritize high-value targets, optimizing the allocation of resources for complex fraud investigations.
3. Analyzing big data to anticipate crime waves
Big data is helping law enforcement move beyond just predicting where the next crime will happen. Instead of only looking at past crime reports, agencies are now analyzing larger trends, like economic downturns and even extreme weather, to anticipate crime waves before they start. It’s a broader approach to helping police prepare for rising threats, rather than just reacting to them.
Example: Research has shown a correlation between extreme weather events and spikes in certain crimes. Law enforcement agencies have begun incorporating climate data and economic trends alongside crime statistics to anticipate surges in theft and fraud following natural disasters, enabling preemptive resource deployment in high-risk areas.
4. Optimizing resources with predictive analytics
Law enforcement agencies don’t just need more resources—they need strategic resource allocation to maximize impact.
Predictive analytics software helps law enforcement go beyond analyzing crime patterns. It enables agencies to:
- pinpoint high-risk areas based on real-time and historical data with crime analysis software. This ensures officers are deployed where crime is most likely to occur
- optimize officer scheduling by predicting demand. This prevents staffing shortages in critical areas and overstaffing in low-risk zones
- prioritize investigations by identifying cases with the highest likelihood of escalation or repeating offenses
Example: Police departments have begun to implement predictive analytics software through crime centers and strategic decision support centers (SDSCs) to improve resource deployment and response times. These centers integrate real-time crime data, predictive models, and more, to optimize patrol strategies and identify high-risk areas.
Predictive Analytics Techniques for Crime Prevention
Predictive analytics isn’t about looking into a crystal ball. It’s about unlocking new possibilities for law enforcement. Crime prevention lies in connecting the dots faster than criminals can adapt. By leveraging AI, machine learning and advanced analytics, agencies have the potential to uncover hidden links between individuals, anticipate where crimes may occur and expose criminal tactics.
Some of the most widely used predictive analytics techniques include:
- Regression Analysis: Identifies relationships between variables to predict future events and crime trends.
- Classification Models: Categorizes data points to detect anomalies and flag suspicious individuals, transactions or behaviors.
- Clustering Algorithms: Groups similar data to uncover patterns, such as links between seemingly unrelated fraud cases or operations being facilitated by organized crime networks.
- Natural Language Processing (NLP): Extracts insights from unstructured text, such as police reports or social media posts.
- Time Series Analysis: Uses historical data to anticipate future trends, such as crime spikes, enabling agencies to prepare for seasonal patterns or emerging threats.
- Decision Trees & Random Forests: Breaks down complex datasets into structured decision pathways to aid in risk assessment and suspect prioritization.
- Neural Networks: Mimics human reasoning by analyzing vast amounts of crime-related data to recognize subtle patterns.
- Geospatial Predictive Analytics: Analyzes location-based data to map high-risk areas, optimize patrol routes and strategically allocate law enforcement resources.
- Sentiment Analysis: Analyzes social media and online conversations to detect sentiment and track potential radicalization trends.
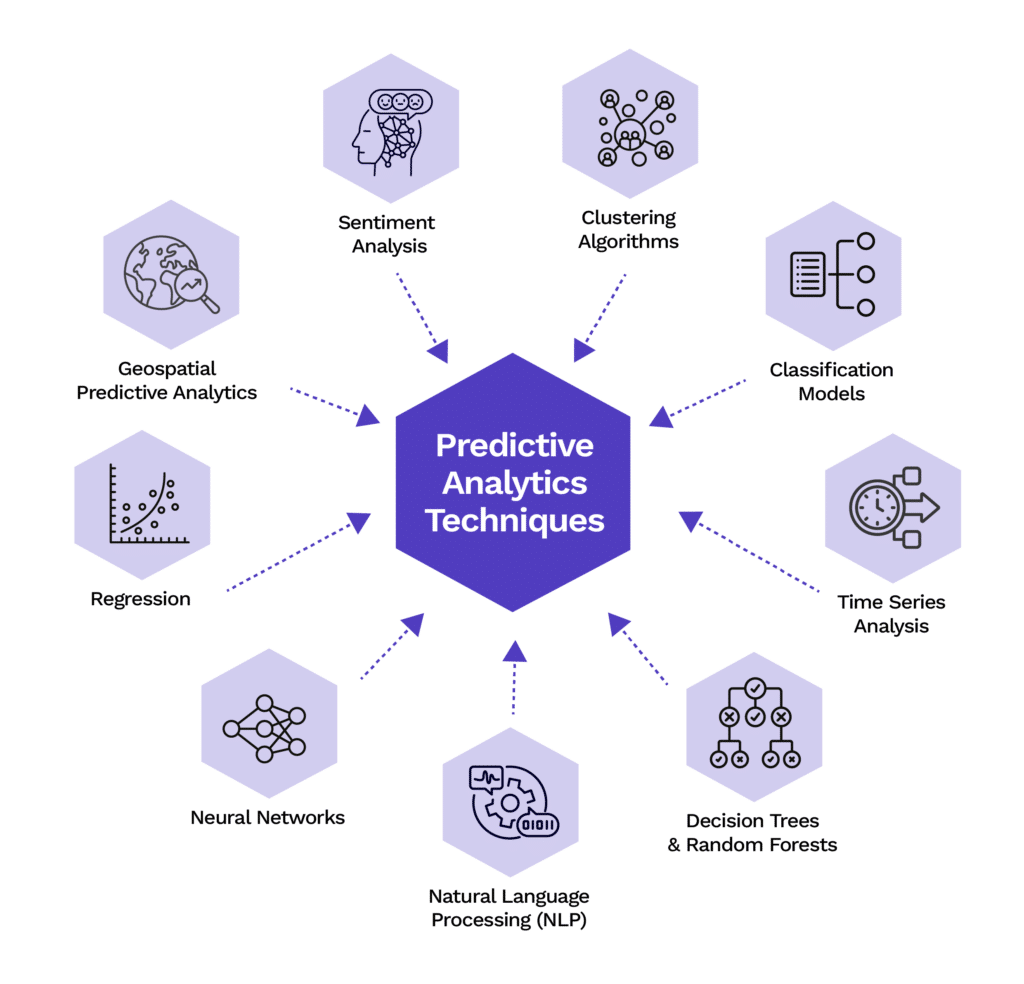
The Benefits and Challenges of Predictive Analytics in Policing
The benefits of predictive analytics include enhanced crime prevention, improved decision-making, better resource allocation and reduced operational costs.
While the benefits are significant, implementing predictive analytics comes with its own set of challenges.
- Data quality and availability – The success of predictive analytics depends on the quality and comprehensiveness of the data. Inconsistent or incomplete data can reduce the accuracy of predictive models.
- Bias in predictive analytics models – Algorithms may inadvertently replicate biases present in historical data, leading to unfair targeting of certain communities. Ensuring fairness and transparency is critical.
- Technical and resource barriers – Implementing predictive analytics software requires significant investment in infrastructure, training and skilled personnel – challenges that can be particularly demanding for smaller departments.
The NEXYTE Advantage: Turning Predictive Analytics into Actionable Decisions
Predictive analytics can be a powerful asset for law enforcement, but without proper application, valuable intelligence may become fragmented, delayed or misused.
NEXYTE, Cognyte’s decision intelligence platform, bridges this gap by converting predictive insights into actionable, data-driven decisions.
- Actionable Insights – Using NEXYTE, agencies can monitor risks and anomalies as they emerge, enabling proactive responses to evolving threats.
- Integrated Intelligence – By automating data fusion, standardizing formats, and enriching incomplete datasets with AI-driven insights, NEXYTE overcomes data quality and availability challenges. This ensures investigators have a unified, contextualized view of threats, reducing blind spots and enabling faster, more confident decision-making.
- Predictive Modeling – NEXYTE’s flexible machine learning framework streamlines the creation and training of predictive models using real data. This eliminates the need for separate solutions and specialized analyst expertise, making advanced analytics more accessible to agencies with limited resources.
- Transparency & Oversight – NEXYTE mitigates challenges such as data quality issues and algorithmic bias by embedding Responsible AI principles. This ensures transparency, fairness, and governance in predictive analytics. By auditing, refining, and validating AI-driven insights, NEXYTE helps law enforcement uphold ethical standards while strengthening community trust.
Learn how NEXYTE turns predictive intelligence into faster, smarter decisions.
The post How Predictive Analytics Software is Transforming Crime Prevention appeared first on Cognyte.
Article Link: Transforming Policing with Predictive Analytics | Cognyte
1 post - 1 participant
Malware Analysis, News and Indicators - Latest topics
Post a Comment
Post a Comment